Why Does NVIDIA Have No Rivals in the AI Chip Industry?
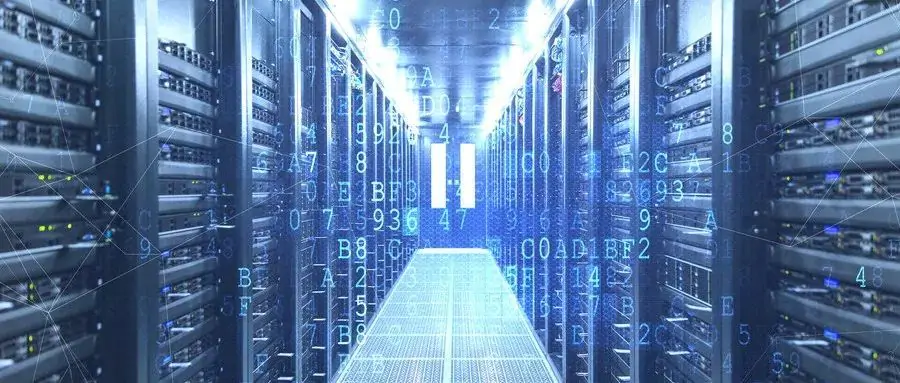
Who is the biggest beneficiary of generative AI? At least in the chip industry, it is undoubtedly NVIDIA, the absolute leader in the GPGPU (general-purpose graphics processing unit) field. In the AI chip and GPGPU fields, who can compete with NVIDIA? The answer is no one.
With the help of outstanding performance, NVIDIA once reached a market value of one trillion US dollars. This is a height that other companies in the chip industry find difficult to reach, and its market value is seven times that of chip giant Intel.
As NVIDIA’s founder, what magic did Jensen Huang use to bring the company to such heights? From Huang’s recent speech at National Taiwan University, we can get a glimpse of it.
“Learning to let go is the core key to success,” Huang said this and did it. Ten years ago, in 2013, Intel was still spending huge sums of money to subsidize mobile tablet manufacturers, while Huawei, with its P6 phone, led HiSilicon’s K3V2 chip to shine. NVIDIA, however, gradually faded out of the booming mobile SoC market at the time.
“Our letting goes paid off, and we created a new market - robotics technology. We have a secure architecture with neural network processors and AI algorithms.” Huang said.
But no one can achieve success solely by “letting go”. In addition to letting go, Huang was more focused. NVIDIA’s achievements come from its focus, its long-term focus on the GPU field, and its catching up with the explosion of the AI ecosystem. These two aspects have made NVIDIA the king of the global chip industry.
The “Madman” Who Knows How to Let Go
Jensen Huang, the founder of NVIDIA, is often referred to as the “madman” in a leather jacket by industry insiders. In this speech, however, Huang departed from his “leather-clad” image and donned a suit, appearing refined and elegant. During the speech, Huang told an interesting story. Ten years ago, Professor Chen from National Taiwan University invited him to visit his physics laboratory, where the entire room was filled with NVIDIA gaming graphics cards inserted into open computers’ motherboards, with large fans mounted on metal frames for cooling. Professor Chen told him, “Mr. Huang, thanks to you, I can accomplish my career.”
Chen’s words deeply impressed Huang, “What he said still moves me today and perfectly embodies the value of our company: to help the Einsteins and Da Vincis of this era accomplish their careers.”
When Einstein was developing the theory of general relativity, he sought the help of contemporary mathematicians.
Today, in this era, whether it is research in AI, physics, or biology, it cannot be separated from the support of computing power. NVIDIA is the leader in AI chips.
“Letting go” and “focus” can be said to be the keys to Huang’s success. Ten years ago, AI was not thriving, and the industry’s focus was on mobile devices. The explosive growth of smartphones and tablets made mobile chips the “battleground” for major chip giants.
NVIDIA was an early player in mobile devices but eventually gave up on the market. According to NVIDIA’s official website, as early as 2008, NVIDIA launched the Tegra chip for mobile devices; in May 2011, NVIDIA acquired Icera to address the shortcoming in baseband, and the latter was a leading innovator in high-performance baseband processors for 3G and 4G networks on smartphones and tablets.
At the time, Huang declared, “This is a critical step for NVIDIA to become a leading company in the mobile computing revolution. By integrating Icera’s technology into Tegra, we will develop an excellent platform to support the best smartphones and tablets in the industry.”
However, NVIDIA failed to win in this round of the mobile computing revolution, with victory going to Apple, Qualcomm, and MediaTek. In 2013, NVIDIA released the Tegra 4 at the International Consumer Electronics Show in Las Vegas, which also became NVIDIA’s “swan song” in the field of mobile chips. Since then, the Tegra series of chips have mainly been used in the Nintendo Switch game console.
AI Chips Lead the Way
Despite the current downturn in the smartphone chip industry, it is still a huge market. Ten years ago, it was very difficult to decide to give up the smartphone chip market.
But Jensen Huang chose to abandon a huge market and create an unknown market. In his speech, Huang exclaimed: “Retreat from the huge smartphone market and create a robot market with an unknown market size. However, we now have a business of billions of dollars in autonomous driving and robotics technology, and we are also creating a new industry.”
In the desktop CPU market, Intel and AMD dominate; in the mobile SoC market, Apple is slightly ahead, with Qualcomm, MediaTek, and RuiChip leading the way. In the AI chip market, there are old chip giants like AMD and Intel, as well as startups like Tenstorrent led by chip guru Jim Keller. Despite the presence of giants and gurus, NVIDIA can still “outshine the rest.”
Why are there no opponents? “NVIDIA wins with CUDA (Compute Unified Device Architecture), wins with software.” A former securities analyst replied to reporters. After interviewing multiple industry experts and GPGPU engineers, CUDA was almost mentioned. The software ecosystem built by CUDA is the key to NVIDIA’s leadership.
How big is NVIDIA’s advantage over other GPGPU vendors? “The difference between an academician and a high school student.” Chip engineer Duke told reporters. And what about AMD? “Academicians and university professors,” he replied.
The key to closing the gap lies in the ecosystem. “The ecosystem comes first, (CUDA) is very similar to the Android system, too mature, too convenient, and the ecosystem is too strong. Like Coca-Cola, programmers are used to this drink (CUDA). And (CUDA) has set a low threshold, like you are bad at math, but you can use a calculator.” Duke explained to reporters.
So can other AI chip companies use tools similar to CUDA? Such as AMD’s ROCm (Radeon Open Compute Platform) and the non-profit organization Khronos Group’s OpenAI.
Duke gave an example to reply: “It’s like buying screws and wrenches from you. You can also not use universal tools, but no one will play with you. AMD also made one, but they don’t even use it themselves.”
Software Ecosystem is Irreplaceable
Senior industry analyst Rogue explained to reporters: “NVIDIA launched CUDA in 2006. It was the launch of CUDA that lowered the barrier to entry for GPU applications. Software developers can use languages like C/C++ through CUDA to write on-chip GPU programs, which reduces the barrier to entry for GPU applications. It was also from that time that GPUs gradually moved away from single-purpose image processing. It can be used not only for image processing but also for high-performance computing.”
In short, CUDA lowered the barrier to entry for GPUs, allowing the application of GPUs to expand from image rendering to various fields, truly becoming a general-purpose processor, hence the term GPGPU (General-Purpose Graphics Processing Unit).
“All of NVIDIA’s architectures are built on CUDA, from the beginning to now, including many levels, such as compilers, debuggers, rich library functions, and various software tools, which are huge resources. If there is a new hardware platform that is not compatible with CUDA, then it means a lot of software porting work for developers. Therefore, some platforms may choose to be compatible with CUDA, which means that CUDA-accelerated software can run on their hardware, but the actual efficiency and performance have yet to be observed, which is also the strength of the CUDA ecosystem.” Rogue added.
On June 5th, an engineer named Jack from a leading GPGPU company told reporters: “After years of development, NVIDIA’s CUDA now has 4 million developers and has formed a monopolistic ecological barrier. The software ecosystem is precisely the most important product competitive factor for downstream customers, which is NVIDIA’s biggest advantage over AMD, Intel, and other startups.”
Since CUDA is so important, can other vendors provide their hardware and use the CUDA ecosystem?
On this issue, Jack believes: “CUDA is a completely closed system. Currently, only AMD can truly be compatible with CUDA, or companies that use CUDA (except NVIDIA itself). AMD and NVIDIA have related IP authorizations, so NVIDIA’s MI series GPGPUs can use CUDA. But other startups cannot use CUDA directly. Currently, there are two approaches for startups. The first is often companies that start their businesses based on AMD, whose chip architecture is similar to AMD’s products, so they can use CUDA directly for hardware conditions. But due to IP issues, they will fine-tune their software stack based on CUDA, which is convenient for users to migrate from the CUDA environment, but there is an IP risk. The second is a completely original software stack, which has the biggest problem that customers have a certain migration cost, which hurts commercial landing.”
Lawrence, CEO of Electronic Innovation Network, also told reporters: “It is not possible for NVIDIA to open up CUDA hardware for other vendors to integrate CUDA into their chips and run their software developed for CUDA. Jensen Huang has completely denied this possibility, after all, CUDA is leading the competition in this area, and NVIDIA cannot open up its advantages to other vendors or even competitors.”
Run! Keep Running No Matter What
In his speech, Jensen Huang addressed the students: “You are about to enter a world undergoing tremendous change, just like when I graduated and encountered the personal computer and chip revolution. You are now at the starting line of AI. Every industry will undergo a revolution and rebirth, so prepare for new ideas. Whether you run for food or to avoid becoming someone else’s food, you often don’t know which situation you are in, but no matter what, keep running.”
NVIDIA began research and development on GPUs very early on and has long focused on GPUs. In the field of AI, what is needed is high computing power parallel computing, which is best suited for GPUs. NVIDIA’s core product is still GPUs, other things have been tried, but later they slowly withdrew.
In addition, NVIDIA’s advantage is not limited to the CUDA ecosystem but also includes hardware architecture and manufacturing processes. Rogue said: “For all chips, hardware architecture is the foundation, like the framework of a house. For example, the H100 uses the latest generation of Hopper architecture, which has some inter-unit collaborative computing for large models and has better acceleration capabilities. Its latest product, the GH200, belongs to a heterogeneous integration architecture, which uses NVIDIA’s own Grace CPU and H100 GPU, and adopts its own NVLink interconnect technology between the CPU and GPU. This architecture solves many data transmission bottlenecks and greatly improves the bandwidth between the CPU and GPU.”
These two products are new products that NVIDIA will soon launch. Currently, the NVIDIA A100 is still the most widely used for large-model training worldwide. Rogue believes: “The A100 still uses the previous generation of the Ampere architecture, which also improves its computing performance throughput for AI, including larger memory and higher bandwidth, which are essential for large-scale computing. We only looked at the latest two generations of architecture. Looking back, NVIDIA evolved from targeting gaming to targeting high-performance computing, generation by generation, which is very important for it.”
Year | Architecture | Process | Process Number of Transistors |
---|---|---|---|
2008 | Tesla | - | - |
2010 | Fermi | 40nm | 3 billion |
2012 | Kepler | 28nm | 7.1 billion |
2014 | Maxwell | 28nm | 8 billion |
2016 | Pascal | 16nm | 15.3 billion |
2017 | Volta | 12nm | 21.1 billion |
2018 | Turing | 12nm | 18.6 billion |
2020 | Ampere | 7nm | 28.3 billion |
2022 | Hopper | 4nm | 80 billion |
Intel once implemented the Tick-Tock strategy (upgrading process technology one year, and upgrading microarchitecture the next year), but it was difficult to continue due to the long delay in the 10-nanometer process. In contrast, NVIDIA has released a total of nine generations of architecture from the Tesla architecture in 2008 to the Hopper architecture in 2022, with even one generation released in less than two years. In addition, due to its close collaboration with TSMC, NVIDIA has always used the most advanced process technology.
Peter, a senior analyst in the TMT industry, told reporters: “The H100 uses TSMC’s 4nm process and integrates 80 billion transistors, which is 26 billion more than the previous generation A100, making it the largest accelerator in the world. Its CUDA core count has skyrocketed to an unprecedented 16,896, which is 2.5 times that of the A100. The floating-point calculation and tensor core operation capabilities have also increased by at least three times. For example, the FP32 has reached 600 trillion operations per second. More importantly, the H100 is designed for AI computing and is optimized for Transformers, with an optimization engine that directly improves the speed of large-scale model training by more than six times. This means that whether training the 175 billion parameters GPT-3 or the 395 billion parameter Transformer model, the H100 can reduce training time from one week to within one day. These breakthrough technological innovations have helped NVIDIA maintain its absolute leadership in the high-end chip market.”
Perhaps, as Jensen Huang said, NVIDIA has been “running all the time”. He told students: “Whatever it is, go after it with all your might, run! Don’t walk slowly.”
Yes, run! Don’t walk slowly. It is through continuous running that NVIDIA has gradually risen to the top of the global chip market. In the GPU field, former leaders 3DX and ATI were successively acquired, but NVIDIA remained standing. In the GPU software ecosystem, Microsoft’s DirectX and ATI Stream emerged, but in this long race, the winner was CUDA.
Perhaps the focus is the reason why NVIDIA can win in the long run. Microsoft’s focus is not on the GPU software ecosystem, and after being acquired by AMD, ATI also tends to focus on heterogeneous collaboration between CPUs and GPUs. This is true for software, and hardware as well. Intel and AMD are leaders across CPUs, GPUs, and FPGAs, while NVIDIA has long focused on GPUs.
Who can challenge Nvidia’s dominance?
Nvidia’s dominance in the chip industry has been established through long-term focus and continuous innovation. In the era of heterogeneous computing, Nvidia has also expanded its product portfolio to include different types of chips. For example, the GH200 chip mentioned earlier combines Nvidia’s own GPU with an ARM-based CPU. In addition, Nvidia has also launched DPU products through acquisitions.
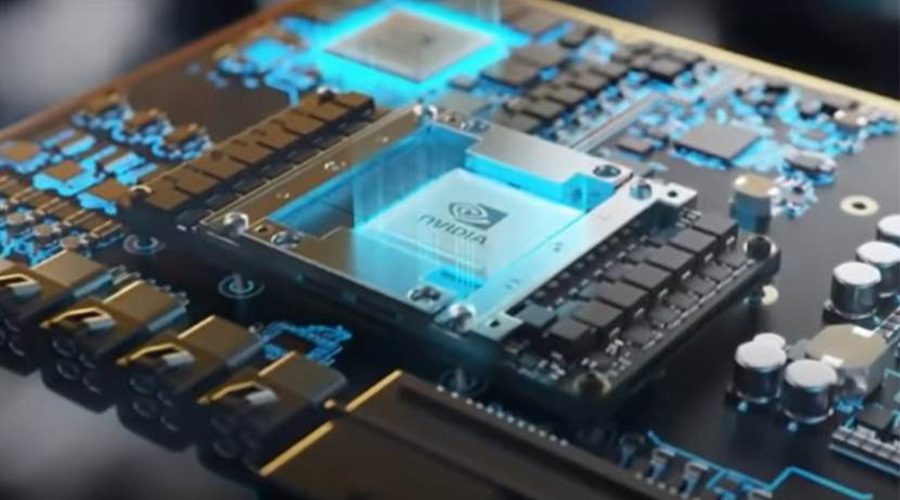
In the first half of 2020, Nvidia acquired Israeli network chip company Mellanox Technologies for $6.9 billion and launched the BlueField-2 DPU later that year, defining it as the “third main chip” after CPU and GPU.
What is a DPU? According to Nvidia’s official website, a DPU is an advanced computing platform for data center infrastructure that provides acceleration for software-defined networking, storage, security, and management services on a large scale.
As data volumes continue to grow, traditional CPUs are unable to keep up with the explosion of data, especially in short videos and visual applications, where the data volume is growing exponentially. DPUs have emerged to solve this problem. Prior to Nvidia’s acquisition of the DPU company, some FPGA manufacturers were also exploring this area. Since then, the DPU market has quickly heated up, with AMD acquiring DPU chip manufacturer Pensando for $1.9 billion in 2022, and several DPU startups emerging in China.
In this wave of “third main chips,” Nvidia is also building its software ecosystem, launching DOCA in 2021.
What is DOCA? Nvidia explains simply and directly: “DOCA is to DPU what CUDA is to GPU.”
“If CUDA is the soul of the GPU, then DOCA is the soul of the DPU. Because if a chip doesn’t have useful software to work with, it’s just hardware, like a phone that can only make calls without a rich ecosystem of apps. It is the software that allows developers to develop a variety of applications, making the hardware applications so rich,” said Rogue.
Through hardware architecture and software ecosystem, Nvidia has built a strong barrier in the GPU field. In the DPU field, Nvidia seems to be replicating this approach.
Who can challenge Nvidia’s position? Currently, there may be no one. “Unless Nvidia makes a major mistake, but the likelihood of that happening is very small,” said Lawrence.
“It is possible for AMD’s MI300 to be the closest product to the H100 in terms of hardware and software ecosystem. Whether it is foreign startups like Graphcore or several domestic companies, there is currently no product that can replace Nvidia’s H100. In addition, Nvidia’s huge shipment volume and investment in chip manufacturing have formed a close partnership with TSMC, rather than a simple customer-supplier relationship. For example, the 4nm process used by the H100 is a specially optimized version based on the public 5nm process developed by Nvidia and TSMC,” said Jack.
Intel was once the leader in the desktop CPU market. However, AMD, under the leadership of Lisa Su, has successfully caught up with Intel. Can AMD also perform miracles in the GPU field? There is indeed a possibility. But the fundamental reason why Intel was caught up by AMD was its wafer manufacturing technology. At that time, Intel was stuck at the 10nm node, but AMD was able to turn the tide through its fabless model and cooperation with TSMC. Nvidia, on the other hand, has a close partnership with TSMC.
Nvidia, which has been running all the time, and Jensen Huang, who has been running all the time, who can overturn them?