Future of AI Hardware: NVLink Switches in DGX H100
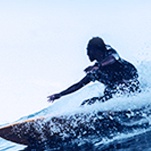
NVIDIA dominates the GPU market with a share of nearly 90% in training and inference tasks. In the DGX H100 SuperPOD architecture, NVIDIA has introduced a higher-speed NVLink solution and utilized both NVLink and InfiniBand's PCIe systems to address communication challenges. While InfiniBand NDR networks are currently mainstream, the architecture of the new NVLink Switch, built upon the H100 hardware, can deliver performance close to doubling that of IB networks in certain AI scenarios. The foundation of AI development lies in increased computational power, and it is expected that achieving ultimate performance while minimizing power consumption will drive future hardware architecture development. Network solutions with speeds exceeding 400G/800G are expected to accelerate deployment.
1. Higher-Speed NVLink Solution in DGX H100 SuperPOD Architecture
Taking NVIDIA, the global leader and benchmark in computational power, as an example, let's make a tentative quantitative assessment under the latest DGX H100 SuperPOD solution to explain why optical networks with speeds exceeding 400G/800G are an inevitable choice in AI frameworks:
(1) NVLink Iteration to Gen4 with a per-channel bandwidth of 100 Gbps
NVLink is a network solution specifically designed for high-speed point-to-point GPU interconnectivity (GPU to GPU). It offers lower overhead compared to traditional networks, where complex network functionalities (such as end-to-end retry, adaptive routing, packet reordering, etc.) can be traded off by increasing the number of ports. Additionally, NVLink-based network interfaces are simpler, allowing application layer, presentation layer, and session layer functionalities to be directly embedded into CUDA itself, further reducing communication overhead.
NVIDIA has met two major requirements for its computational solutions through the iteration of four generations of NVLink between 2016 and 2022 (a dedicated interconnect solution for specific needs). This has allowed GPUs to achieve the highest possible performance by using specialized protocols and system designs. While traditional PCIe Gen5 provides only 32Gbps per channel, NVLink offers a per-channel bandwidth of up to 100Gbps, with multiple channels connecting the GPU system. The latest NVLink 4 has upgraded from 12 links in the previous generation to 18 links, providing each GPU with a bidirectional bandwidth of 900GB/s (7200Gbps).
When combined with the NVIDIA H100 GPU:
1) The DGX H100 server houses eight H100 GPUs.
2) Each H100 GPU is connected to the internal NVSwitch3 chip through 18 NVLink4 connections (each server is equipped with four NVSwitch3 chips).
3) Each NVLink4 has 2 lanes, with each lane operating at 100Gbps-per-lane (x2@50Gbaud-PAM4). This translates to a one-way bandwidth of 200Gbps or 25GB/s, and a bidirectional bandwidth of 50GB/s. With 18 NVLink4 connections, the H100 GPU can achieve a bidirectional bandwidth of 900GB/s.
(2) NVSwitch Chip Iteration to Gen3 with Each Chip having 64 NVLink-4 Interfaces
In addition, NVIDIA has released the NVSwitch chip, designed for internal use in supercomputing servers, which functions similar to a switch ASIC. It further connects multiple GPUs together at high speeds through the NVLink protocol interfaces mentioned above. In the generation of the H100 chip and NVLink 4 protocol, the NVSwitch 3 chip solution is introduced, manufactured using TSMC's 4nm process. It enables point-to-point connections between GPUs and incorporates an ALU to provide NVSwitch with a computational throughput of 400GFLOPS in FP32. Each NVSwitch 3 chip features 64 NVLink 4 interfaces.
According to technical documentation, the NVSwitch 3 chip has a size of 50mm*50mm and includes a SHARP controller capable of parallel management of up to 128 SHARP groups. The embedded ALU helps NVSwitch provide a computational throughput of 400GFLOPS in FP32 and supports various precision calculations such as FP16, FP32, FP64, and BF16. Additionally, the PHY interface is compatible with 400Gbps Ethernet or NDR InfiniBand connections. Each cage has four OSFP interfaces for NVLink4, and it also supports FEC verification. The NVSwitch 3 chip provides 64 NVLink4 interfaces, with each NVLink4 channel operating at x2, resulting in a one-way bandwidth of 200Gbps. A single chip can provide a one-way bandwidth of 64 x 200Gbps = 12.8Tbps (1.6TB/s), or a bidirectional bandwidth of 3.2TB/s.
2. Solving Architectural Challenges with NVLink and PCIe Solutions
(1) Basic Principles
The communication between GPU cards is based on NVLink, while the communication between CPU/storage and inter-cluster communication is based on PCIe. In NVIDIA's DGX H100 server, each server is equipped with 8 H100 GPUs and 4 NVSwitch 3 chips, all interconnected. Alongside the server release, NVIDIA also introduced NVLink switches that incorporate 2 NVSwitch 3 chips, forming the NVLink network together with the GPU servers and NVLink 4 protocol.
(2) DGX H100 Server Architecture
In the Motherboard Tray, the ConnectX-7 network cards play a crucial role in the network infrastructure, and they are based on the PCIe solution. According to publicly available specifications, each server is equipped with 8 ConnectX-7 InfiniBand/Ethernet adapters (400Gb/s).
NVLink switches are an innovation in the H100 system and a highlight of the application of 800G optical communication solutions. NVIDIA has released a new NVLink switch designed in a compact 1U size with 32 OSFP interfaces. Unlike regular switches, each NVLink switch is equipped with 2 NVSwitch3 chips, providing a total of 128 NVLink4 interfaces (64 NVLink4 interfaces per NVSwitch3). The switch offers a bidirectional bandwidth of 6.4TB/s (200Gbps per NVLink4 in one direction, resulting in a one-way bandwidth of 25.6Tb/s with 128 x 200Gbps).
The introduction of NVLink switches aims to build computational clusters for the H100 SuperPOD. According to NVIDIA's design, each SuperPOD system consists of 32 servers, equivalent to 256 H100 GPUs, delivering AI performance of up to 1EFlops. Each system is equipped with 18 NVLink switches, providing a bidirectional bandwidth of 57.6TB/s. Additionally, the 400Gb/s ConnectX-7 network cards in the 32 DGX H100 servers of each system are connected to IB switches externally, facilitating the connection of multiple SuperPOD systems.
(3) Two-Layer NVSwitch Chip Design
The first layer of the NVSwitch chip is located within the servers, while the second layer is within the switches. There are 128 L1 layer chips (32 servers with 4 chips each) and 36 L2 layer chips (18 NVLink switches with 2 chips each). The interconnection of all 256 GPUs within a SuperPOD is achieved independently through the NVLink protocol and NVLink switches, bypassing the CX7 PCIe network.
From a communication network perspective, the essence of the high computational power and high throughput upgrade in the DGX H100 SuperPOD lies in externalizing the NVLink, previously used for efficient GPU connections within servers, to the entire cluster. With the help of the new NVLink switches, a two-layer network (L1 and L2) is established to enable GPU-to-GPU connections across servers and cabinets.
3. Network Architecture and Predicted Optical Module Requirements
In the latest H100 architecture calculation, for 8 nodes (single server), 18 pairs of NVLink connections are required, along with 36 OSFP interfaces, which translates to 36 units of 800G optical modules. If an InfiniBand network is needed, a traditional leaf-spine dual-layer architecture is employed, requiring 800G or 2x400G (NDR) modules. The quantity relationship is similar to a regular cluster and can be calculated separately based on different scales.
4. Conclusion
In the latest NVLink Switch architecture of the NVIDIA DGX H100 SuperPOD, the GPU+NVLink+NVSwitch+NVLink switch architecture requires a large number of 800G communication connections. The NVLink system corresponds roughly to a 1:4-1:5 ratio of GPUs to 800G optical modules, while the IB NDR network requires even more.
Specifically, NVIDIA holds a market share of nearly 90% or more in the GPU training and inference segment. With the introduction of higher-speed NVLink solutions in the DGX H100 SuperPOD architecture and the utilization of both NVLink and InfiniBand's PCIe systems to address communication challenges, the new NVLink Switch architecture, built upon the H100 hardware foundation, can deliver performance gains close to or even double that of the IB network in certain AI scenarios. The development of AI relies on improved computational power, and it is expected that maximizing performance while minimizing power consumption will be the main driving force behind future hardware architecture advancements. Network solutions with speeds exceeding 400G/800G are expected to be deployed at an accelerated pace.
In the latest NVLink Switch architecture of the H100, the calculation shows that for a single server with NVLink, 18 pairs of connections and 36 OSFP interfaces are required, which translates to 36 units of 800G optical modules. For a cluster of 32 servers, such as a SuperPOD, it would require 36x32=1152 units of 800G optical modules. If the NVLink Switch architecture is not utilized or there is a need for multi-cluster expansion, the InfiniBand NDR network can be used. In that case, a traditional leaf-spine dual-layer architecture with an 800G+2x400G (NDR) solution is required. The quantity relationship can be referenced from a regular cluster, with the key being the significant increase in system bandwidth. Additional calculations can be made based on different scales.
Due to the limited supply of H100 GPUs, there is some disparity in the market's understanding of their actual architecture. However, the high-speed optical modules and technologies such as CPO/LPO/MPO indicate that the main direction of future hardware architecture development is to pursue high performance under extremely low power consumption. The overall efficiency of the system's computational power has a "bucket effect," where the network component is more prone to becoming a bottleneck, impacting various training and inference considerations. Therefore, the iteration of high-speed optical networks is a crucial requirement for AI.