DCC Technology for AIGC Network Solution
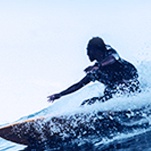
In 2023, we witnessed the comprehensive rise of AI artificial intelligence technologies, represented by AIGC large models such as ChatGPT and GPT-4. These models integrated multiple functionalities such as text generation, code development, and poetry creation, showcasing remarkable content generation capabilities that left a profound impact. As an IT professional, the communication technology behind AIGC large models deserves deep consideration. Without a robust network, the training of large models would be impossible to discuss. To build large-scale training model clusters, not only GPU servers and network cards are required as fundamental components, but also the network infrastructure needs to be addressed. What kind of powerful network supports the operation of AIGC? How will the comprehensive advent of the AI wave revolutionize traditional networks?
The aforementioned AIGC large models are impressive not only because they are fed with massive amounts of data but also due to continuous advancements in algorithms. Furthermore, the human computing power has reached a certain level of development. The powerful computational infrastructure is fully capable of supporting the computational demands of AIGC.
When training large-scale models, the model size often exceeds the memory and computational capacity of a single GPU, necessitating the utilization of multiple GPUs to distribute the workload. There are three methods for distributing GPU workloads during large model training: tensor parallelism, pipeline parallelism, and data parallelism.
In practical deployment, the design of the network must take into account the bandwidth and latency requirements imposed by these parallel strategies to ensure efficient and effective model training. Sometimes, these three parallel strategies are combined to further optimize the training process. For example, a large-scale model might employ data parallelism across multiple GPUs to process different subsets of data, while also utilizing tensor parallelism within each GPU to handle different portions of the model.
With the continuous advancement of large models, the demand for computational power in model training has been increasing and doubling approximately every three months. The GPT-3 model, for example, had 175 billion parameters, trained on 45TB of training data, and consumed 3640 PFlops/s-Days of computational power. ChatGPT3, which utilized 128 A100 servers with a total of 1024 A100 cards for training, required 4 100G network channels per server node. However, for other large models like ChatGPT4 and ChatGPT5, the network requirements would be even higher.
As AIGC has developed, the number of model parameters for training has skyrocketed from billions to trillions. To complete training on such a massive scale, the underlying support of GPUs has also reached the scale of tens of thousands.
The biggest factor that affects GPU utilization is the network.
As a computing cluster consisting of tens of thousands of GPUs, there is a great need for high bandwidth when it comes to data interaction with storage clusters. Additionally, during training computations in a GPU cluster, parallelism is employed, resulting in a significant amount of data exchange between GPUs, which also requires substantial bandwidth.
If the network is not robust and data transfer is slow, GPUs have to wait for data, leading to decreased utilization. Reduced utilization prolongs training time, increases costs, and diminishes user experience.
The industry has conducted research to determine the relationship between network bandwidth throughput, communication latency, and GPU utilization. The resulting model is illustrated in the following diagram:
The stronger the network throughput capacity, the higher the GPU utilization rate. Conversely, as communication latency increases, GPU utilization decreases. It can be said that a robust network is the foundation for large models.
What kind of network can support the operation of AIGC?
To address the high network requirements of AI cluster computing, the industry has proposed multiple solutions. In traditional strategies, we commonly encounter three technologies: InfiniBand, RDMA, and chassis switches.
1. InfiniBand Networking
For professionals familiar with data communication, InfiniBand networking is well-known. It is considered the best approach to building high-performance networks, ensuring high bandwidth, congestion-free communication, and low latency. In fact, InfiniBand networking is used in networks supporting models such as ChatGPT and GPT-4. However, this technology has the drawback of high cost, several times higher than traditional Ethernet networking. Additionally, InfiniBand is relatively closed, with only one mature vendor in the industry, limiting user choices.
2. RDMA Networking
RDMA, or Remote Direct Memory Access, is a novel communication mechanism. In an RDMA solution, data can directly communicate with the network card, bypassing the CPU and complex operating systems. This significantly improves throughput and ensures lower latency.
Initially, RDMA was primarily deployed in InfiniBand networks. Now, it has gradually been ported to Ethernet. The current mainstream networking solution is based on the RoCE v2 protocol, which builds RDMA-capable networks. However, technologies like PFC (Priority Flow Control) and ECN (Explicit Congestion Notification) in this solution, although designed to avoid link congestion, can lead to frequent triggering of sender pauses or throttling, affecting communication bandwidth.
3. Chassis Switches
Some foreign internet companies have hoped to meet high-performance network demands with chassis switches. However, this solution faces challenges such as limited scalability, high power consumption, and large failure domains. Therefore, it is only suitable for small-scale AI cluster deployments.
4. Next-generation AIGC Network: DDC Technology
Given the limitations of traditional solutions, a new solution called Distributed Disaggregated Chassis (DDC) has emerged. DDC "disaggregates" traditional chassis switches, enhancing scalability and allowing flexible network design based on the size of the AI cluster. Through this innovative approach, DDC overcomes the limitations of traditional solutions, providing a more efficient and flexible network architecture for AI computing.
From the perspectives of scale and bandwidth throughput, DDC fulfills the networking requirements of AI large-scale model training. However, network operation involves more than just these two aspects; it also requires optimization in terms of latency, load balancing, management efficiency, and other factors. For this purpose, DDC adopts the following technical strategies:
(1) VOQ+Cell-based forwarding mechanism to combat packet loss
When the network encounters burst traffic, it may overwhelm the receiving end, leading to congestion and packet loss. The VOQ+Cell-based forwarding mechanism used by DDC effectively addresses this issue. The specific process is as follows:
Upon receiving a data packet, the sender classifies and stores it in the VOQ. Before sending the data packet, the NCP (Network Control Processor) sends a Credit message to confirm whether the receiver has sufficient buffer space. The data packet is sliced into cells and dynamically load balanced to Fabric nodes only when the receiver confirms processing capability. If the receiver is temporarily unable to process, the data packet is temporarily stored in the sender's VOQ without immediate forwarding.
This mechanism makes full use of caching, significantly reducing or even avoiding packet loss. As a result, it improves overall communication stability, reduces latency, and enhances bandwidth utilization and business throughput efficiency.
(2) PFC single-hop deployment to completely avoid deadlock
PFC (Priority Flow Control) is used for flow control in lossless RDMA networks. It creates multiple virtual channels on Ethernet links and assigns priorities to each channel. However, PFC can cause deadlock issues.
In the DDC network architecture, since all NCPs (Network Control Processors) and NCFs (Network Control Fabrics) are considered as a single device, there are no multi-level switches, completely avoiding PFC deadlock problems.
(3) Distributed OS for enhanced reliability
In the DDC architecture, management functions are centrally controlled by the NCC (Network Control Chassis). However, this approach carries the risk of a single point of failure. To mitigate this issue, DDC employs a distributed OS, enabling each NCP and NCF to independently manage and have separate control and management planes. This not only greatly improves system reliability but also facilitates deployment.
Conclusion
Through its unique technical strategies, DDC not only meets the networking requirements of large-scale AI model training but also optimizes various details to ensure stable and efficient network operation under complex conditions.